In today’s digital world, financial institutions face a big threat from cybercrime. They lose about $600 billion each year, which is 0.8% of the world’s GDP. Fraud attempts jumped by 149% in the first quarter of 2021 compared to the year before. This rise is mainly because of more online transactions during the COVID-19 pandemic.
But, there’s good news. The financial services industry has found a strong ally against fraud – artificial intelligence (AI). In 2022, more than half of financial institutions used AI to fight fraud. They see its huge potential.
AI systems are great at handling lots of data quickly and accurately. They do better than old software in spotting normal and fake customer actions. AI uses complex algorithms to learn from past data and keep up with new fraud patterns. This means less need for people to help make models to fight fraud.
AI in banking also speeds up fraud detection. It can quickly spot and mark unusual transactions, app use, payment methods, and other financial actions. This fast action helps banks stay ahead of clever fraudsters.
Key Takeaways
- AI systems can handle lots of data quickly and accurately, cutting down on mistakes in spotting normal and fake customer actions.
- AI uses complex algorithms to learn from past data and adjust to new fraud patterns, needing less human help.
- AI in banking reduces false positives, making customer experiences better while keeping things secure.
- AI is good at finding different types of banking fraud, like identity theft, phishing, credit card theft, and fake documents.
- AI fraud detection systems watch for suspicious activities 24/7, catching them right away.
Understanding the Growing Need for AI Fraud Detection
Online transactions have grown fast, leading to more fraud. Financial fraud losses could hit $10 billion in 2023. Globally, 26% of people fell victim to scams or identity theft from 2022 to 2023, losing $1 trillion.
Old fraud detection methods can’t keep up with cyber threats. This makes advanced solutions more urgent than ever.
Fintech AI tools are a promising answer. AI fraud detection uses advanced algorithms to spot fraud quickly. It gets better over time, adapting to new threats.
Citibank cut phishing attacks by 70% with natural language processing. Walmart reduced shoplifting by 25% with real-time video analysis.
AI fraud detection algorithms have cut eCommerce fraud by up to 96%. By 2022, over half of financial institutions used this technology. As fraud attempts surge, strong digital banking security is key.
“For every dollar lost to fraud, the U.S. financial services sector faces an additional $4.23 in costs.”
The global economy loses $600 billion to cybercrime each year. With generative AI, fraud losses could hit $40 billion in the U.S. by 2027. The financial industry must use AI to fight fraud.
AI in Financial Services: Core Components and Benefits
The financial services industry is quickly adopting AI to improve customer service, make operations smoother, and keep finances safe. At the heart of these changes are several important parts that work together. They bring real benefits to banks and other financial institutions.
Key Components
AI in finance starts with collecting lots of data, creating useful features, and training models. These steps help AI systems understand and learn from big data. They can spot fraud patterns and alert banks quickly to keep money safe.
Primary Benefits
- AI makes processing financial data faster and more accurate.
- It helps catch fraud in real-time, building trust with customers.
- Machine learning finds new fraud trends, keeping finances secure.
- Customers enjoy smoother transactions with fewer errors.
- AI helps banks handle more transactions without needing more staff.
By using AI, banks can achieve great things. They can work better, serve customers better, and keep finances safe. This change is happening now, with leaders seeing AI’s huge potential to lead their companies forward.
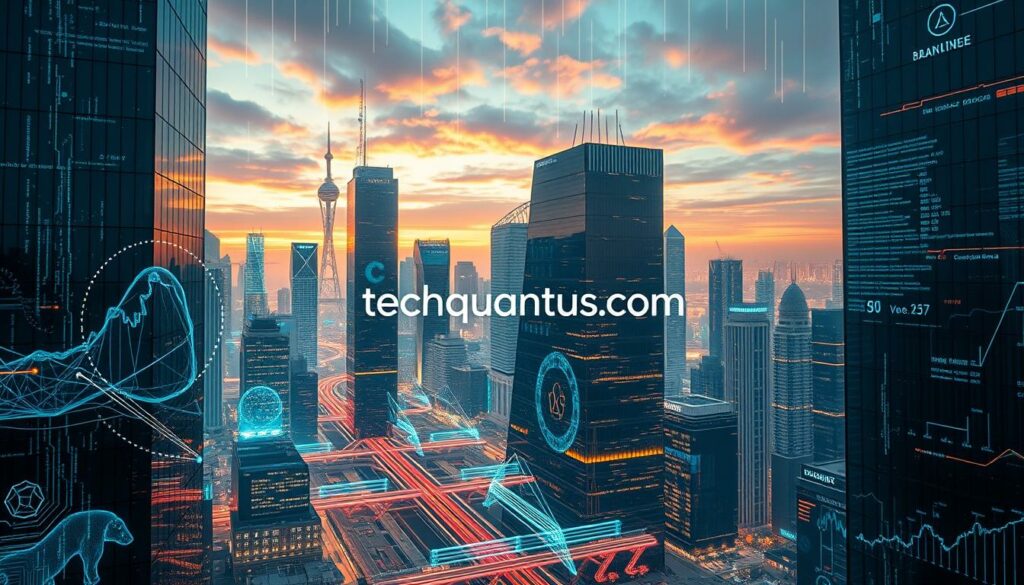
“AI is revolutionizing the financial services industry, enabling banks to streamline processes, enhance customer experiences, and fortify financial security like never before.”
Essential Building Blocks of AI Fraud Detection Systems
AI fraud detection systems in finance need key parts to work well. High-quality, diverse data is at the heart. This data helps train and improve AI models. Getting the data ready is vital for AI to spot patterns correctly.
Feature engineering is also crucial. Data scientists pick and polish data points to boost AI’s predictive power. This step is key to finding what makes fraud different from real transactions.
These systems use both supervised and unsupervised learning. Supervised learning uses labeled data, while unsupervised learning finds oddities in data without labels. The success of these systems relies on data quality, diversity, and feature engineering to spot fraud and cut down on false alarms.
By focusing on these key elements, banks and financial institutions can build AI fraud detection systems. These systems are more precise, quick, and effective in fighting fraud.
Ensuring Data Quality and Diversity
Good, varied data is the base for training AI models well. Banks must work hard to gather a wide range of data. This includes:
- Combining data from various sources, like transaction records and customer profiles, to get a full picture of finance.
- Keeping data fresh to keep up with new fraud and customer habits.
- Using checks to find and fix data problems like missing values and oddities.
Optimizing Feature Engineering
Feature engineering is a big deal for AI fraud detection systems. Data scientists need to pick and polish the right data points. This helps AI tell fraud from real transactions. This process includes:
- Looking at transaction data to find patterns and oddities that might show fraud.
- Adding data from outside, like customer info and trends, to make the feature set better.
- Keeping the feature engineering process sharp as fraud schemes and customer habits change.
By focusing on these key parts, banks can make AI fraud detection systems better. These systems will be more accurate, quick, and good at fighting fraud.
Types of Financial Fraud AI Can Detect
Artificial intelligence (AI) is a big help for banks fighting fraud. It can spot identity theft, phishing attacks, credit card fraud, and more. AI systems are very good at finding and stopping these crimes.
Common Fraud Patterns
AI looks at lots of data to find fraud patterns. For identity theft, it watches for odd account changes and login attempts. It also checks emails for phishing attacks by looking at the content and sender.
For credit card fraud, AI checks spending patterns and predicts future spending. It can also check signatures and spot forgeries, helping to stop document fraud.
Detection Mechanisms
AI learns from big datasets to get better at finding fraud. It uses supervised learning to learn from examples of fraud and real transactions. Unsupervised learning finds odd activities without examples.
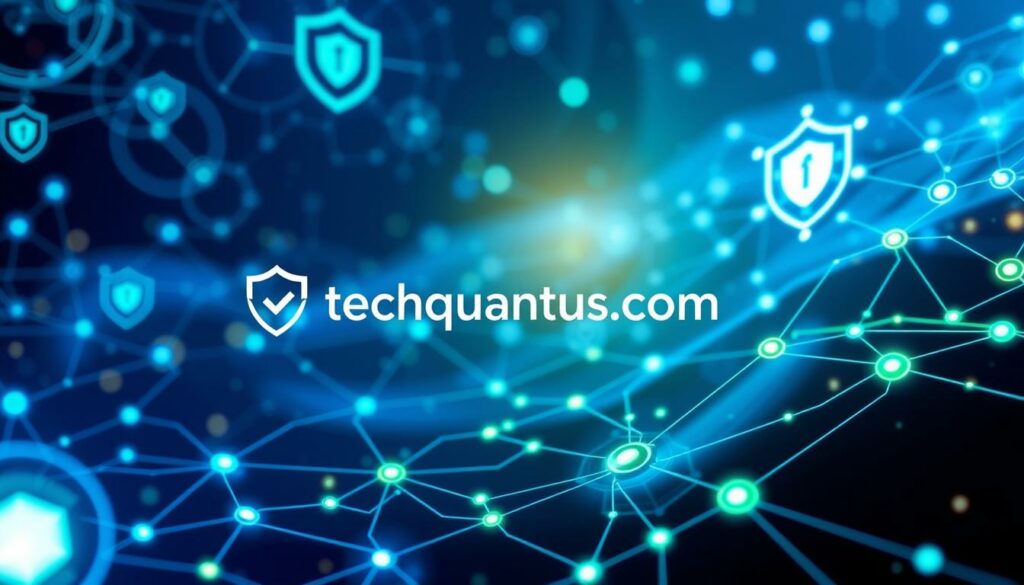
AI uses natural language processing, predictive analytics, and anomaly detection. These tools help it quickly find threats in huge amounts of data. This is much faster than old methods.
Fraud Type | AI Detection Mechanisms |
---|---|
Identity Theft | Monitoring unusual account activities, credential changes, and suspicious login patterns |
Phishing Attacks | Analyzing email content, sender information, and communication patterns to identify suspicious messages |
Credit Card Fraud | Detecting anomalies in spending patterns and using predictive modeling to identify potential fraudulent transactions |
Document Forgery | Authenticating signatures, spotting forgeries, and differentiating between original and fake identities |
By using AI, banks can fight fraud better. This helps protect customers and reduces the harm of financial crimes.
Implementing Real-Time Detection and Monitoring
In today’s fast-changing digital world, real-time fraud detection and transaction monitoring are key for financial security. AI-powered security systems help analyze data quickly. They spot and stop suspicious activities fast.
These systems check lots of data fast, keeping fraud at bay. They act quickly to protect money and prevent losses. They get better over time, keeping up with new fraud methods.
Real-time transaction monitoring uses AI to watch transactions and user behavior. It finds and stops unusual activities right away. This keeps everything running smoothly and safely.
Good fraud detection saves money by cutting down on costs. It reduces the need for investigations and legal fees. It also stops many types of fraud, keeping everything secure.
As the fintech market grows, so does the need for strong fraud detection. Using these advanced tools, banks can protect their customers and stay safe from fraud.
Metric | Value |
---|---|
Financial institutions in the UK aim to respond to transaction monitoring alerts within 2-3 seconds | Significant enhancement in fraud detection for instant payments |
Real-time transaction monitoring provides milliseconds to make decisions on transaction legitimacy | Emphasizes the need for quick action to prevent potential fraud |
Automated transaction monitoring systems can analyze vast amounts of data in real-time | Allows for swift identification of suspicious patterns and potential fraud |
Automated transaction monitoring systems help reduce manual workload | Streamlining alert management and quickly releasing false positives, leading to improved operational workflow and fraud detection capabilities |
Integrating AI and machine learning in real-time transaction monitoring | Enhances fraud detection accuracy by rapidly analyzing transaction data to identify patterns and anomalies indicative of suspicious activity |
By using real-time fraud detection and monitoring, banks can outsmart fraudsters. They protect their customers and keep their finances safe in a changing world.
Machine Learning Models for Fraud Prevention
Machine learning (ML) algorithms are key in fighting financial fraud. They help financial institutions create strong fraud prevention systems. These systems keep up with new fraud tactics.
Supervised Learning Approaches
Supervised learning models use labeled data to learn from. They look at past data to spot fraud patterns. For example, Citibank cut phishing attacks by 70% with machine learning.
Unsupervised Learning Methods
Unsupervised learning finds odd patterns in data without labels. It catches fraud threats that rule-based systems miss. Walmart cut shoplifting by 25% with machine learning video analysis.
Using both supervised and unsupervised learning helps fight fraud better. Supervised models handle known fraud, while unsupervised finds new threats.
ML algorithms, predictive modeling, and anomaly detection help financial institutions stay ahead of fraud. These tools are crucial in protecting the financial system as fraud tactics evolve.
“Machine learning algorithms got up to 96% accurate in cutting fraud for eCommerce, a University of Jakarta study found.”
By using ML algorithms, financial institutions can stop fraud before it starts. This keeps customers’ trust and security. As the industry grows, using these technologies will be key in fighting fraud.
Overcoming Implementation Challenges
Putting AI-powered fraud detection systems in financial services has big challenges. Making sure data is good and available is key. It’s also important to follow data privacy laws and rules. Also, making sure the system works well with old systems can be hard.
Another big problem is false positives. This means good customer actions are seen as bad. It can make customers unhappy and hurt trust in the AI. Keeping up with new fraud ways means the AI needs to keep getting better.
- Maintaining data quality and privacy compliance
- Integrating AI systems with legacy infrastructure
- Minimizing false positives to preserve customer experience
- Regularly updating AI models to adapt to new fraud patterns
To beat these challenges, we need a plan that covers everything. This includes good data handling, smooth system integration, and keeping the AI sharp. With these steps, banks can use AI to stop fraud and keep customers happy.
Challenge | Percentage of Importance |
---|---|
Infrastructure-related | 99% |
Implementing Machine Learning Models | 1% |
“Ninety-nine percent of the challenges in implementing AI/ML in financial institutions are infrastructure-related, whereas 1% is related to implementing machine learning models.”
By tackling these main challenges, banks can make the most of AI for fraud detection. They can also give customers a smooth experience while fighting off new fraud tricks.
Measuring Success and ROI of AI Fraud Detection
Financial institutions are turning to AI for fraud detection. It’s key to measure how well these systems work and their return on investment (ROI). By looking at metrics like fewer fraud cases and less financial loss, we can see how effective AI is.
Calculating ROI means comparing the cost of AI to the savings it brings. This helps understand the financial benefits of using AI for fraud prevention.
Good AI fraud detection means fewer false alarms and quicker detection. It also builds trust with customers. Over time, it can lead to less manual checks and better compliance with rules.
To really see how AI is doing, set clear goals and track important data. This way, you can tell if AI is worth the investment.
But, figuring out AI’s ROI can be tough. It involves looking at both obvious and hidden benefits. Things like happier employees and more satisfied customers are important too.
Keeping an eye on AI’s performance and working with others is key. This ensures that investments in AI fraud detection are wise and effective.
FAQ
What is the global economic cost of cybercrime?
Cybercrime costs the global economy $600 billion each year. This is 0.8% of global GDP.
How much have fraud attempts increased in Q1 2021 compared to the previous year?
Fraud attempts went up by 149% in Q1 2021 compared to the year before.
What percentage of financial institutions have adopted AI for fraud detection in 2022?
Over half of financial institutions used AI for fraud detection in 2022.
How does AI enhance fraud detection capabilities?
AI makes fraud detection better by processing lots of data quickly. It also reduces mistakes in spotting fraud. Machine learning learns from past data, adapting to new fraud patterns.
What percentage of participants in the 2023 Currents report by DigitalOcean increased cybersecurity spending on advanced security software?
The 2023 Currents report by DigitalOcean shows 37% of participants boosted spending on advanced security software.
What are the key components of AI in financial services for fraud detection?
Key parts of AI in financial services include collecting data, engineering features, training models, and detecting anomalies. It also involves continuous learning and alerting systems.
What are the primary benefits of AI-powered fraud detection in financial services?
AI fraud detection boosts efficiency and accuracy in handling large data sets. It detects fraud in real-time and adapts to new threats. It also improves customer experience by reducing false positives.
What are the essential building blocks for effective AI fraud detection systems?
Essential blocks include high-quality, diverse data for training ML models. Effective data preparation and feature engineering are also key. AI systems use supervised and unsupervised learning approaches.
What types of financial fraud can AI detect?
AI can spot identity theft, phishing, credit card fraud, and document forgery. It watches for unusual account activities and checks email patterns for phishing. It also analyzes spending patterns for credit card fraud and authenticates signatures.
How can AI systems implement real-time fraud detection and monitoring?
AI systems analyze transaction data and user behavior in real-time. They flag suspicious activities immediately. This allows for quick action to prevent losses.
What are the different machine learning approaches used for fraud prevention?
Supervised learning uses labeled data to train models for fraud detection. Unsupervised learning finds new fraud patterns in unlabeled data. Both are important for preventing fraud.
What are the key challenges in implementing AI fraud detection systems?
Challenges include ensuring data quality and privacy, integrating with existing systems, and managing false positives. Keeping up with threats requires continuous updates. Overcoming these needs careful planning and ongoing model optimization.
How can the success of AI fraud detection be measured?
Success is measured by reducing fraudulent transactions and financial losses. It’s also about improving detection accuracy. ROI is assessed by comparing costs to prevented losses and efficiencies. Success indicators include lower false positives and faster detection times.
Source Links
1 . Fraud detection using AI in banking | Infosys BPM
2 . Understanding AI Fraud Detection and Prevention Strategies | DigitalOcean
3 . How AI and Machine Learning Can Combat Financial Fraud
4 . Generative AI is expected to magnify the risk of deepfakes and other fraud in banking
- The Impact of 5G on Cloud Computing and IT Infrastructure
- Measuring ROI in IT Marketing: Key Metrics to Track
- Digital Marketing Automation Tools for IT: A Complete Guide
- Why Content Marketing is Essential for IT Companies
- How to Set Up a Virtual Firewall for Your Cloud Infrastructur